On-Demand Webinar
2025 Cloud AI Risk Report: Helping You Build More Secure AI Models in the Cloud
- AI
- Cloud
- Research
- Tenable Cloud Security
Join us to uncover critical cloud security vulnerabilities, misconfigurations, real-world risks, and expert strategies for securing AI workloads.
The explosive growth of AI and large language models (LLMs) in the cloud has introduced unprecedented cloud security challenges, from unpatched AI software vulnerabilities to misconfigured cloud environments that expose sensitive data. Are your AI workloads secure?
Get help addressing this challenge. Join Tenable AI and cloud security experts for this on-demand webinar, exploring key insights from the 2025 Tenable Cloud AI Risk Report and offering practical strategies for securing AI models in the cloud.
Topics covered will include:
- Key report findings observed in self-managed AI developer tools and frameworks offered by AWS, Google Cloud, and Microsoft Azure
- Mitigation and security best practices for protecting your AI workloads and applications
- Effective approaches for leveraging Tenable Cloud Security to secure your AI workloads
Who should attend?
Security leaders, cloud architects, DevSecOps, and infosec professionals responsible for securing AI workloads and applications in public cloud environments.
Register and watch on-demand now.
Click here to review the webinar transcript:
Franklin Nguyen
Hi, everyone. My name is Franklin Nguyen. I'm a product marketer here at Tenball. And I support our Tenable Cloud Security solution. Joining me today is Damien Lim. Damien, would you like to introduce yourself and give a little background about yourself?
Damien Lim
Yes, thank you, Franklin. I just had to unmute myself. Hi, my name is Damien. I am the AI evangelist here at Tenable. Really great to be here and excited to share, you know, this topic with everyone.
Franklin Nguyen
Awesome. Thanks, Damien. And of course, Damien is the star of the show. So I'll lead off and transition to Damien where he will provide some insights.
Franklin Nguyen
So with that, let's go ahead and dive right in. I kind of gave it away a little bit, but today these are some of the things that we'll be covering. We're going to talk about some of the trends in a I as well as some of the challenges we're going to highlight. Some of the risks that we identified from the cloud risk report that we published this year, as well as providing you the audience with best practices.
Franklin Nguyen
And again, before I proceed further, I do want to acknowledge that your time is valuable and we appreciate you joining us today for this webinar. So we hope that you find it useful to your ongoing efforts within your organization.
Franklin Nguyen
So with that, why don't we go ahead and begin. So we're going to level set real quick, right. I think there are maybe there may be perceptions about what we're going to be covering today in terms of secure UN quote securing AI. So what I'm going to do is first establish what we will be talking about in terms of. A I risks and how to secure them.
Franklin Nguyen
So first let's establish what we're going to be talking about. A I cloud services and related solutions used by organizations, right. So we have this notion of just broad A I inventory within an organization. The three broad areas are A I workloads, so cloud native, cloud native A I services provided by a WS Azure GCP. The storage of data used to train models that you may be using or building in your organization and finally related to that a I software that you may be that you may have installed on workloads as well. So these are not collectively exhaustive, but these are three areas that we will be touching on over the course of this webinar.
Franklin Nguyen
And I would like to add that although this is the main topic, we will also touch upon end user safety measures that we can provide. And what I mean by that is if users in your organization are using. End user solutions like ChatGPT and inputting potentially sensitive information into it. There are ways that we can kind of surface that as well.
Franklin Nguyen
So jumping into the thick of it now, what are we seeing? Not just us, but using other analyst reports. Here we see McKinsey conducted a survey of organizations and their usage of a I. As you can tell, things are moving up and to the right. And they are growing more rapidly over time. I don't think this is a novel concept. I believe that over the course of the past few years, there is a growing need to leverage a I to build better products, gain better insights. And I think this survey lends to that notion.
Franklin Nguyen
Double clicking a bit further, this is some data that we derived from our research team. So over the course of two years looking at production workloads that our customers of our customers I should say, the research team found that a lot of organizations are using cloud native services to help refine or develop their own A I models and. Solutions or even integrate AI into their existing solutions. As you can see, it's covered across all the major major service, major cloud service providers, Azure, AWS and GCP. And generally speaking, if for those unaware, these services typically allow organizations to either incorporate existing foundation models into their solutions. Or use these services to build, train and deploy LLMs and other AI services.
Damien Lim
No, I I think this is a good general overview. Obviously what's covered is, you know, might not be exhaustive here, but this, you know, highlighting certain key data points, right, from a threat research report perspective. So this is very insightful. Thanks frankly for sharing.
Franklin Nguyen
Thanks, Damien. So before we go into the next slide, I'd like to take a quick pause and provide a quick survey. Your response is greatly appreciated. So let me go ahead and launch that.
Franklin Nguyen
Here we go. I've just launched it. So again, your response would be greatly appreciated. I'll give it about a minute for responses and then we'll go ahead and move forward.
Damien Lim
It will be really interesting I think Franklin to, you know, see how the respondents, you know, having different sorts of challenges. So excited to see the poll come up and and it looks like there's a. Pretty even spread, but there's two that stand out. Misconfigurations and data exposure. I think those are typical. Nothing that I find surprising in that regard. Does anything jump out at you, Damien, from the results so far? I I wouldn't say like something that's really. Peculiar and this is aligned to, you know, my thought process as well. In fact, as part of my presentation, I'll be focused on some of these very burning issue, if you will, based on the poll results. So happy to share a little bit about, you know, our findings specific to those.
Franklin Nguyen
Awesome. And I think we can wrap it up. I again, I appreciate your response, everyone. I'll go ahead and stop sharing the poll. And I'll share the results so you can see that. OK, Can you see the results?
Damien Lim
I think so.
Franklin Nguyen
OK, so let's go ahead and jump in. So, oops, that's weird. OK, so using new and different services can introduce a risk. So the idea is, you know, Pandora's box is open. And with new technologies such as AI come great responsibility, right? As organizations adopt new technology, there's that learning curve that takes place where they need to understand how to best implement and use this new technology. But inherent in that are potential new risks related to that and over time. Organizations are able to better able to secure and prevent themselves and prevent those risks. But these are things that all organizations need to account for.
Franklin Nguyen
So what do I mean by this, right? So let's go ahead and there we go. So let's start with this notion here. So we have this Jenga puzzle here. Just think of this as your organization. And within your organization, as you deploy workloads in the cloud, in this instance, leveraging AI to create models that you can use internally or externally for customers, there's broad types of risks that organizations need to be aware of.
Franklin Nguyen
And what are these risks? First are obviously critical vulnerabilities. Publicly, public access to these workloads, over privileged access and misconfigurations. As you can tell, these are not new risk types and also they're not collectively collectively exhaustive, right? And there's a lot of overlap between them. But these are common risks that we have seen that we will share in more detail in the report.
Franklin Nguyen
Additionally, we have this notion of a toxic combination, meaning that a collection of these individual risks tied together. Can pose an even greater threat to an organization that will allow a threat actor to, for example, access a publicly accessible workload which has a A I library installed in it with a critical vulnerability that also has access to an S3 bucket that has sensitive information. Now that collection of different risks tied together. Can allow that that actually to not only exfiltrate the sense of data, but also conduct either a ransomware attack or do other nefarious things to compromise the organization, leading to this instance where you're left vulnerable, right?
Franklin Nguyen
With this mindset, I'd like to hand it off to Damon where he will. Put more meat behind each of these different types of risks and what we found in the report.
Damien Lim
All right, awesome. Thanks Franklin for that. So let's kick off with the A I specific vulnerabilities. And so from the threat research report findings, right #1 is that we found that 70% of A I workloads had at least one critical vulnerability vulnerability. Versus 50% in non A I workloads. So why is that? It's because you know, A I workloads rely heavily on open source components such as, you know, Pytorch or Tensorflow. So that makes them, you know, inherently vulnerable to unpatched security flaws. Now unlike you know, traditional workloads, A I models often incorporate multiple libraries. As well as frameworks and dependencies and if that's left unpatched, you know could expose organizations to significant security risk. And you know this could range from, you know, data corruption to the insertion of backdoors into a I models.
Damien Lim
Next. And so, so if you look at you know our report, right, we analyzed. A high severity vulnerability in call. That's the CVE 202338545 and this is related to a heap buffer overflow and this remain unpatched for over a year. So leaving the critical A I infrastructure at risk and with that the consequences, you know attackers could easily exploit this vulnerability to gain unauthorized access. Extract model data or even tamper with the AI training pipelines.
Damien Lim
Next. And if you if you take a different example and this one is with the Chuan Hu ChatGPT CVE 20243234 and this one is categorized as a critical vulnerability and this actually allowed. Attackers to steal sensitive files because the application used an outdated vulnerable iteration of the Gradio open source Python package. So I think the key take away here is, you know, AI workloads introduce unique vulnerabilities that organizations must monitor and patch just like any other critical infrastructure enterprise application and the dependencies for them.
Damien Lim
And what's interesting is, you know, I think in people's mind when you talk about a I security, and I think this goes back to the poll earlier, right. We often think about model integrity, but data exposure, right, is an even bigger risk. So I'm glad that, you know, folks have noticed that as well. And it's, you know, operationally something that everyone's concerned with, right, because A I as everyone knows. Right. The those workloads rely on massive data sets and if that data is not secured, it can lead right to intellectual, intellectual property theft, compliance violations and regulatory fines. But before we dive in a little bit more into these findings, for those who are not familiar, I just want to kind of help with the definition. So the A WS Bedrock is focused on generative A I using the. Pre-built foundational models. So if you think about like Cloud, Llama and so on and so forth, this is working through an API without having you know organizations to manage the infrastructure or train your own model. So this is a great service that a lot of organizations are tapping into.
Damien Lim
Now with that said, I would say that one of the most common risks that we found is storage misconfiguration. So in Amazon Bedrock 14.3% of training data storage has the block public access feature disabled. And this means that, you know, confidential model training data could be accidentally exposed to the internet or create an opportunity to poison its data.
Damien Lim
Next. And furthermore, overprivileged policies are another major concern. In Bedrock, 5% of organization organizations have at least one over permissive bucket. So if the attacker gains access right to an A I storage bucket with weak permissions, they could do things like steal the training data and use it to replicate, you know, proprietary models of their own, inject poison data to manipulate you know your the A. decision making process, or even delete or modify AI training data sets, and that would obviously lead to a skewed model output and you know create that unexpected outcome.
Damien Lim
And then lastly, in the AWS SageMaker, we found that 90% of organizations have left the SageMaker Notebook instance With root access enabled and hopefully everyone knows that this is pretty mind-blowing and the finding. So with root access users can manipulate exfiltrate AI model and the IP behind it and data in S3 buckets and kind of like anticipating a you know a question ahead of time would be what is Amazon Sagemaker. So this is a fully managed. Machine learning platform that helps developers and data science, you know, very quickly build and train and deploy, you know, this ML models at scale. And I think something to keep in mind is that and and we have to reiterate, right, the AI models are built on data sets and you know, typically they are very sensitive and they have, you know, proprietary attributes to it. And so if you think about like customer data sets, a financial model or otherwise, exposing this data could be devastating, right, for any organization. So the key take away would be organizations must treat AI training data as a critical asset, enforcing strict access controls and ensuring that no data sets are left vulnerable to exposure.
Damien Lim
And then with that, Franklin, if you would do me a big favor, if you can push out this poll. So this is the second poll. We like to get a quick pulse on, you know, how confident are you in your organization's ability to secure a I workloads in the cloud? Are you confident, somewhat confident, not confident or unsure? So go ahead and make your selection. I'll be appreciate that and I think. The results here would set us up very nicely as we review the best practices for securing AI workloads next.
Franklin Nguyen
So I see numbers are coming in. It looks like we have a most of the respondents are looking at they're somewhat confident. So everyone is concerned they are working on securing that but. At least, you know, folks on the on in this meeting, right. Very transparent, right. Being honest that you know there are some gaps and hopefully, you know, we can address some of them and you know, give you some recommendations.
Damien Lim
Yeah, definitely. Nothing too surprising here.
Franklin Nguyen
Good, honest feedback. I I would be surprised if someone was extremely confident in their. Security measures. I don't think you can always, I don't think you can always be secure enough, right? There's always going to be new risks and exposure to organizations, right? So it's a cat and mouse game. And also I use this term a lot, whack-a-mole effort, right? Because again, in the cloud, new risks always pop up and it's a matter of how to best prioritize. And focus on the risks that matter the most and then work your way down there, right? Because organizations don't have infinite resources at their disposal to tackle all the risks. So you need to have a solid approach to tackling key issues.
Franklin Nguyen
So with that, it looks like a roughly even spread with somewhat confident leading the way followed by don't know, which is fair cause. You know, the audience may not be in this security area for your organization or it may not be close enough to the the A I team for example and not confident is third on that. So hopefully now Damien, I'll go ahead and pass that back off to you. I'll go ahead and jump to the next slide where you can share some of some of the best practices that we have. Provided in the report.
Damien Lim
Yep, absolutely. Thanks, Franklin. All right. So now we have identified, you know, some of the key risk in AI workloads mentioned earlier. And you know, I would like to thank everyone that participated in the poll. I think this is very a great, you know, eye-opener even for myself, right, learning that, you know, organizations out there and you know, folks are still struggling with trying to close the gap. So let's walk through, you know, five of the essential best practices, right, to secure them. And the first is, hopefully obvious, is gain unified visibility right across your A I workloads. The thing is, you know, you can't really secure what you can't see. So I think that's a good first step. You know, many organizations lack full visibility into their A I usage and A I development across their environments. So really the solution here is to deploy, you know, A I security posture management or A ISPM and related security tools, right to monitor, you know, A I specific resources.
Damien Lim
Then moving on to the next, I would say that you know, apply least privilege access controls are just as important. Why? Because you know, we've seen overprivileged service accounts are one of the biggest security gaps in a I workloads. So I think it's you know really important for us to limit access to a I models and the training infrastructure following the familiar zero trust approach that you know and security right that that's something that we are very focused on and and zeroed in.
Damien Lim
And then for the next one I would say securing A I training data and storage. Is also something that is a huge concern because you know if you have a misconfigured storage, this is just an easy target right for attackers. And if everyone remembers right, you know deep seek definitely made a huge splash right in terms of disrupting the LLM space here and but if I don't know if everyone can remember when they were launched in January. Very quickly we discovered that data, DeepSeek had a data leak and Riz actually found that it exposed sensitive information including chat history, secret keys and back end details. So that's definitely a lesson to be learned. Obviously they they did mitigate that, but you know that is a serious consequence, right? And that's a real world example, so. I think it's important for us to always enforce strict access policies, disable public sharing on all AI related data.
Damien Lim
And then moving on, I would say that number four, something for us to really, really focus on is, you know, prioritizing the AI specific vulnerabilities in terms of remediation, right. We know that traditional vulnerability management does not necessarily cover AI specific threats. So the solution I would say is to implement, you know, a I risk detection to identify unpatched CV ES in machine machine learning frameworks. Some examples I brought up earlier was Pytorch and Tensorflow. And further definitely consider a system that can enable teams or you yourself to be more strategic by prioritizing the mitigation of these vulnerabilities.
Damien Lim
And then I would say #5 is enforce and secure your cloud configurations, right? Cloud security misconfigurations or default configurations as I laid out earlier in A I services right can cascade into major security incidents. So the advice here would you know, you know, continuously monitor and remediate any non-compliant A I infrastructure discovered in. You know the various cloud environments that you work in, be it a WS, Azure or GCP.
Damien Lim
And then lastly, I would like to just say that this is not exhaustive in terms of the best practices discussed in today's webinar. Additional guidelines can be found in the Cloud A I Risk report, so feel free to produce that resource which will provide a link at the end of this presentation.
Damien Lim
So I would say the key takeaway is AI security must be proactive, addressing misconfigurations and vulnerabilities, and then, you know, assessing your risk before they are exploited.
Damien Lim
And lastly, a note on Tenable solution and how we can help, right? Tenable One is an AI powered exposure management platform. One important key aspect is that it provides full visibility into exposures across your attack surface, including emerging emerging A I because we seamlessly integrate into vulnerability management and cloud security technologies. So this combination of AI SPM found in our cloud security as well as A I aware found in vulnerability management solutions. gives you the 360 degree visibility to effectively manage your risk for both shadow AI and in-house AI LLM model development locally as well as in the cloud.
Damien Lim
So as we wrap up, I would like to kind of leave you with this. AI is already transforming how we operate in the modern world, I'm sure. You know you you see that day in day out either through productivity or what have you and and you know making our lives much more, you know easier, right in many, many sense. So but I think it also introduces right a new attack surface and this fast moving risk landscape. So securing these A I workloads isn't just a technical requirement but it's actually a business imperative. At Tenable, we believe that visibility, accountability must evolve alongside innovation. And with the right tools and approach, you can empower your teams or yourself, right, to innovate securely and safely.
Franklin Nguyen
So with that, we'd love to hear from you. So if you've got any questions about anything we've covered, please definitely drop them in the Q&A chat window. And while we review these questions, you'll find some recommended next steps listed on the screen. Oh, by the way, if we don't get to your questions today, we'll definitely follow up with you directly after this session. So let's go ahead and dive in.
Damien Lim
So OK, I'll I see this question on I guess our own internal best practices, right with tenable also leveraging A I within your solution. How are you addressing this risk? So the two things I would mention here #1 is. In terms of A I usage within the tenable organisation, we do have a A I, A I governance council and this really helps us with steering the organisations to use sanction A I apps. So that's one I would say and this is a, you know it's a cross domain participation across you know our company. So all these stakeholders would provide input, feedback and review on the A I tools that we use. So that's one aspect. We also have an InfoSec team that use variety of security tools to monitor a I usage and a I LLM development as well. And what's really interesting as you know, I I think that's a common saying, right? You know, we eat our own food, right? And so the technologies and solutions I mentioned earlier, AISPM, AI aware is something we actively use within our own InfoSec team to report on, you know, if we see something that is off and then they'll take actions on that. So that that's, you know, a couple of ways we can think about it.
Franklin Nguyen
OK, yeah, I think most of the questions related to the webinar have been answered and others somewhat related, we can provide responses to as well. After the webinar, yeah. OK. Yeah, let me answer one more frankly, if you don't mind.
Damien Lim
So I think there was a question that came in, you know, do you have a process in place for prioritizing A I related vulnerabilities like the one I mentioned CVE 202338545 and how do you handle long unpatched risk in A I packages? So I I think that's a great question as well. I would say that A I packages often lack in patching because they are. embedded in containers or pipelines. And so Tenable's approach is to really help teams identify these packages and then prioritize them based on exploitability. And for the unpatched issues, we recommend implementing compensating controls. Hopefully, you know, people are familiar with things like, you know, network segmentation. I also kind of mentioned this earlier, you know, applying, you know, strict access management, runtime monitoring and I think it's also really important, you know, for folks to consider establishing, you know, backup and recovery of protocols dealing with this particular issue.
Damien Lim
And then I would say very quickly the last one here, what are the top open source LLMS? From an adoption perspective, maybe this is where I I talked about Deepseq earlier. So I I know that's a huge interest. There's a huge interest in you know what other open source LLMs are out there besides Deepseq. If you do have a concern using that, I would also consider things like Llama from Meta AI that seems to be popular GPT4 Turbo from Open AI. Claude from Anthropic and then lastly Gemma from Google. So these are some of the more popular ones for you to consider if you are, you know, in the journey of building AI applications on LLMs. So those are good ones.
Franklin Nguyen
There you go. Yeah. So again, thanks everyone for your time today. I know everyone is quite busy, but we hope that you found the insights and findings from this report useful to you. We also hope that you'll be able to see the report in full where you'll be able to glean more insights than we shared today. Additionally, I want to say that. In general, again, the the risks shared today conceptually at a high level are not novel in nature. Misconfigurations and vulnerabilities are always going to be there, but being able to methodically work through what we like to say here at Tenable is organizations need to have visibility first and foremost that. Damian mentioned you cannot secure what you cannot see. So being able to see everything in your organization across your entire organization, whether it's on Prem or in the cloud, from there being able to surface all risks but also prioritize them and then finally providing remediation efforts, right. So following that. Easy to understand, but more likely difficult to implement process will allow you to enhance your organization's security posture. And we believe that Tenable offers the right solution with Tenable One to provide you with that ability both on Prem and in the cloud.
Franklin Nguyen
I think we've. Answered most of the questions. Any of the other ones we can take offline. Again, we understand that your time is valuable and we hope that you did find this webinar useful. Anything else, Damien, on your end?
Damien Lim
No. Again, you know, to echo Franklin, you know, really appreciate everyone, you know, stopping by and hope that everyone learned something today. I appreciate your participation and you know, let's continue to engage. If you are, if you're curious about getting a demo, we just didn't have enough time. But if you're interested about the A I Aware or AISPM, feel free to take a look at the links we provide on the screen. And if you want a demo, you can do so on our website. So again, appreciate everyone on the call. Hopefully you took something valuable and it's been an honour to share this discussion with everyone. Thanks everyone. Take care.
Speakers
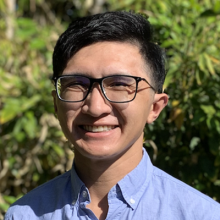
Franklin Nguyen
Principal Product Marketing Manager, Tenable
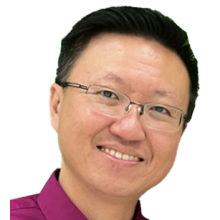
Damien Lim
Senior Product Marketing Manager, Tenable